Deep Art Gallery 🧠
Neural Style Transfer, ConvNet(VGG19), Transfer Learning, tf-gpu
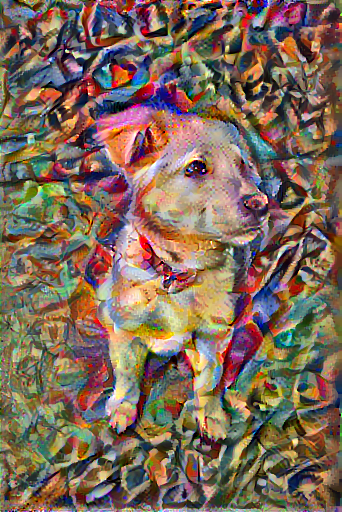
.jpg)
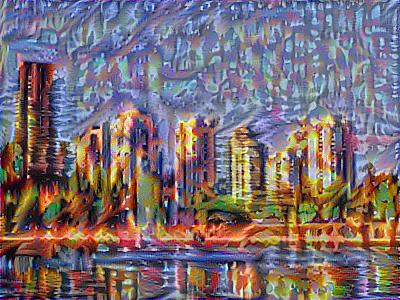
.jpg)
.jpg)
.jpg)
.jpg)
.jpg)
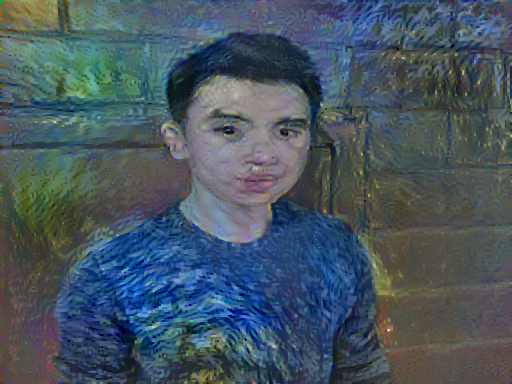
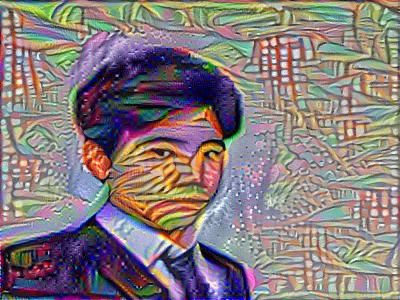
Neural Style Transfer works by choosing a content image and a style image and then 'drawing' the content image using style of the style image.
In implementation, all we are doing is calculating some derivatives to make a number small as possible.
This is the cost function we are trying to minimize. As $J(GeneratedImage)$ gets smaller, we get the art we want. Think of cost function as distance from our art being beautiful. G is initialized as a random noise image. We will use Adam optimization to compute the gradient. Think of gradient as small step towards prettiness.
So every iteration, G will be subtracted with gradient of $J(GeneratedImage)$ slowly becoming beautiful.
Content Cost Function $J_{content}(C,G)$
$$J_{content}(C,G) = \frac{1}{4 \times n_H \times n_W \times n_C}\sum _{ \text{all entries}} (a^{(C)} - a^{(G)})^2\tag{1} $$
- Here, $a$ stands for activation of the lth layer in our convNet.
- $n_H$, $n_W$, $n_C$ is the dimension of the layer. (Height, width, depth).
- The constants in front are just for normalization.
Style Cost Function $J_{style}(S,G)$
$$J_{style}^{[l]}(S,G) = \frac{1}{4 \times {n_C}^2 \times (n_H \times n_W)^2} \sum _{i=1}^{n_C}\sum_{j=1}^{n_C}(G^{(S)}_{(gram)i,j} - G^{(G)}_{(gram)i,j})^2\tag{2} $$
- The constants in front are just for normalization
- The gram is a function that just calculates the correlation between horizontal vectors in the given matrix(which is our depths)
- We will calculate gram of activation layer from both content and generated layer for all combinations of depths(i,j).
- And this is just one layer. Then we compute for all layers. This is why it takes so long to generate our image.
- Note that the picture below 'unrolled' a 3d volume into 2d matrix.
- As you can see style cost function is less straightforward. "If you don't understand it, don't worry about it" - Andrew NG.
content_image = load_img('images/newby.jpg')
style_image = load_img('images/kandinsky.jpg')
plt.subplot(1, 2, 1)
imshow(content_image, 'Content Image')
plt.subplot(1, 2, 2)
imshow(style_image, 'Style Image')
def tensor_to_image(tensor):
tensor = tensor*255
tensor = np.array(tensor, dtype=np.uint8)
if np.ndim(tensor)>3:
assert tensor.shape[0] == 1
tensor = tensor[0]
return PIL.Image.fromarray(tensor)
content_layers = ['block5_conv2']
style_layers = ['block1_conv1',
'block2_conv1',
'block3_conv1',
'block4_conv1',
'block5_conv1']
num_content_layers = len(content_layers)
num_style_layers = len(style_layers)
def vgg_layers(layer_names):
""" Creates a vgg model that returns a list of intermediate output values."""
# Load our model. Load pretrained VGG, trained on imagenet data
vgg = tf.keras.applications.VGG19(include_top=False, weights='imagenet')
vgg.trainable = False
outputs = [vgg.get_layer(name).output for name in layer_names]
model = tf.keras.Model([vgg.input], outputs)
return model
style_extractor = vgg_layers(style_layers)
style_outputs = style_extractor(style_image*255)
#Look at the statistics of each layer's output
# for name, output in zip(style_layers, style_outputs):
# print(name)
# print(" shape: ", output.numpy().shape)
# print(" min: ", output.numpy().min())
# print(" max: ", output.numpy().max())
# print(" mean: ", output.numpy().mean())
# print()
def gram_matrix(input_tensor):
result = tf.linalg.einsum('bijc,bijd->bcd', input_tensor, input_tensor)
input_shape = tf.shape(input_tensor)
num_locations = tf.cast(input_shape[1]*input_shape[2], tf.float32)
return result/(num_locations)
class StyleContentModel(tf.keras.models.Model):
def __init__(self, style_layers, content_layers):
super(StyleContentModel, self).__init__()
self.vgg = vgg_layers(style_layers + content_layers)
self.style_layers = style_layers
self.content_layers = content_layers
self.num_style_layers = len(style_layers)
self.vgg.trainable = False
def call(self, inputs):
"Expects float input in [0,1]"
inputs = inputs*255.0
preprocessed_input = tf.keras.applications.vgg19.preprocess_input(inputs)
outputs = self.vgg(preprocessed_input)
style_outputs, content_outputs = (outputs[:self.num_style_layers],
outputs[self.num_style_layers:])
style_outputs = [gram_matrix(style_output)
for style_output in style_outputs]
content_dict = {content_name:value
for content_name, value
in zip(self.content_layers, content_outputs)}
style_dict = {style_name:value
for style_name, value
in zip(self.style_layers, style_outputs)}
return {'content':content_dict, 'style':style_dict}
extractor = StyleContentModel(style_layers, content_layers)
results = extractor(tf.constant(content_image))
# print('Styles:')
# for name, output in sorted(results['style'].items()):
# print(" ", name)
# print(" shape: ", output.numpy().shape)
# print(" min: ", output.numpy().min())
# print(" max: ", output.numpy().max())
# print(" mean: ", output.numpy().mean())
# print()
# print("Contents:")
# for name, output in sorted(results['content'].items()):
# print(" ", name)
# print(" shape: ", output.numpy().shape)
# print(" min: ", output.numpy().min())
# print(" max: ", output.numpy().max())
# print(" mean: ", output.numpy().mean())
style_targets = extractor(style_image)['style']
content_targets = extractor(content_image)['content']
image = tf.Variable(content_image)
def clip_0_1(image):
return tf.clip_by_value(image, clip_value_min=0.0, clip_value_max=1.0)
opt = tf.optimizers.Adam(learning_rate=0.02, beta_1=0.99, epsilon=1e-1)
style_weight=1e-2
content_weight=1e4
def style_content_loss(outputs):
style_outputs = outputs['style']
content_outputs = outputs['content']
style_loss = tf.add_n([tf.reduce_mean((style_outputs[name]-style_targets[name])**2)
for name in style_outputs.keys()])
style_loss *= style_weight / num_style_layers
content_loss = tf.add_n([tf.reduce_mean((content_outputs[name]-content_targets[name])**2)
for name in content_outputs.keys()])
content_loss *= content_weight / num_content_layers
loss = style_loss + content_loss
return loss
@tf.function()
def train_step(image):
with tf.GradientTape() as tape:
outputs = extractor(image)
loss = style_content_loss(outputs)
grad = tape.gradient(loss, image)
opt.apply_gradients([(grad, image)])
image.assign(clip_0_1(image))
train_step(image)
train_step(image)
train_step(image)
tensor_to_image(image)
Do 1000 iteration and save every 200th iteration image
import time
with tf.device("/gpu:0"):
start = time.time()
epochs = 10
steps_per_epoch = 100
step = 0
for n in range(epochs):
for m in range(steps_per_epoch):
step += 1
train_step(image)
print(".", end='')
display.clear_output(wait=True)
display.display(tensor_to_image(image))
print("Train step: {}".format(step))
# save current generated image in the "/output" directory
imageio.imwrite("output/" + str(2*100) + ".png", tensor_to_image(image))
end = time.time()
print("Total time: {:.1f}".format(end-start))
imageio.imwrite("output/" + str(2*100) + ".png", tensor_to_image(image))
def high_pass_x_y(image):
x_var = image[:,:,1:,:] - image[:,:,:-1,:]
y_var = image[:,1:,:,:] - image[:,:-1,:,:]
return x_var, y_var
x_deltas, y_deltas = high_pass_x_y(content_image)
plt.figure(figsize=(14,10))
plt.subplot(2,2,1)
imshow(clip_0_1(2*y_deltas+0.5), "Horizontal Deltas Original")
plt.subplot(2,2,2)
imshow(clip_0_1(2*x_deltas+0.5), "Vertical Deltas Original")
x_deltas, y_deltas = high_pass_x_y(image)
plt.subplot(2,2,3)
imshow(clip_0_1(2*y_deltas+0.5), "Horizontal Deltas Styled")
plt.subplot(2,2,4)
imshow(clip_0_1(2*x_deltas+0.5), "Vertical Deltas Styled")
plt.figure(figsize=(14,10))
sobel = tf.image.sobel_edges(content_image)
plt.subplot(1,2,1)
imshow(clip_0_1(sobel[...,0]/4+0.5), "Horizontal Sobel-edges")
plt.subplot(1,2,2)
imshow(clip_0_1(sobel[...,1]/4+0.5), "Vertical Sobel-edges")
def total_variation_loss(image):
x_deltas, y_deltas = high_pass_x_y(image)
return tf.reduce_sum(tf.abs(x_deltas)) + tf.reduce_sum(tf.abs(y_deltas))
tf.image.total_variation(image).numpy()
total_variation_weight=30
@tf.function()
def train_step(image):
with tf.GradientTape() as tape:
outputs = extractor(image)
loss = style_content_loss(outputs)
loss += total_variation_weight*tf.image.total_variation(image)
grad = tape.gradient(loss, image)
opt.apply_gradients([(grad, image)])
image.assign(clip_0_1(image))
image = tf.Variable(content_image)
import time
with tf.device("/gpu:0"):
start = time.time()
epochs = 10
steps_per_epoch = 100
step = 0
for n in range(epochs):
for m in range(steps_per_epoch):
step += 1
train_step(image)
print(".", end='')
display.clear_output(wait=True)
display.display(tensor_to_image(image))
print("Train step: {}".format(step))
end = time.time()
print("Total time: {:.1f}".format(end-start))
file_name = 'generated_image.png'
imageio.imwrite("output/" + 'generated_image' + ".png", tensor_to_image(image))
References:
The Neural Style Transfer algorithm was due to Gatys et al. (2015). The pre-trained network used in this implementation is a VGG network, which is due to Simonyan and Zisserman (2015). The whole code is basically from tensorflow website listed below with little changes(to save images and use gpu)
- Leon A. Gatys, Alexander S. Ecker, Matthias Bethge, (2015). A Neural Algorithm of Artistic Style
- Harish Narayanan, Convolutional neural networks for artistic style transfer.
- DeepLearningAi(Coursera) (2020). Deep Learning Specialization
- TensorFlow (2019). Neural style transfer